TUTORIALS
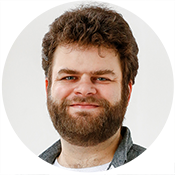
David GARCIA
Complexity Science Hub Vienna, Austria
Analyzing complex social phenomena through social media data
David Garcia has been a group leader at the Complexity Science Hub Vienna since September 2017. The aim is to build a research group funded by WWTF (Vienna Research Groups for Young Investigators Call). He holds computer science degrees from Universidad Autonoma de Madrid (Spain) and ETH Zurich (Switzerland). David did a PhD and Postdoc at ETH Zurich, working at the chair of systems design.
David’s research focuses on computational social science, designing models and analysing human behaviour through digital traces. His main work revolves around the topics of emotions, cultures, and political polarization, combining statistical analyses of large datasets of online interaction with agent-based modeling of individual behaviour. His thesis “Modeling collective emotions in online communities” provides an example of how agent-based modeling can be used to construct an integrated approach to collective emotional phenomena in cyberspace. His postdoctoral research on opinion polarization and online privacy has been funded by the Swiss National Science Foundation and the ETH Risk Centre.
David’s work lies at the intersection of various scientific disciplines, combining methods from network science, computer science, and statistical physics to answer questions from psychology, economics, and political science. His interdisciplinary collaborations span more than 50 co-authors in 12 countries. David has published more than 20 journal articles, 15 conference papers, and five book chapters, and serves as reviewer for prestigious journals and as program committee member of numerous computer science conferences.
-
The wealth of data generated by our digital society, when combined with computational methods like agent-based modeling and natural language understanding, provides a new window to study human behavior at new scales and resolutions. This enables the analysis of complex social phenomena in which temporal dynamics and network structures require the use of large and detailed data. I will present an overview of complex social phenomena that have been analyzed through social media data, one of the most accessible and powerful data sources in our digital society. First, I will show how social media data can be used to analyze collective emotions and their long-term effects in terms of solidarity. Second, social media data can capture states of multidimensional polarization that can be explained by cognitive science models. Third, social media data can capture gender inequality across countries and illustrates the role of positive externalities, also known as network effects. And fourth, I will show how the presence of intelligent technologies in online platforms generate the phenomenon of complex privacy, by which the individual decision to share data with an online platform is affected by the decisions of others.
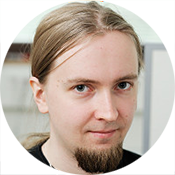
Mikko KIVELA
Aalto University, Finland
Multilayer Networks
I am a network scientist and an assistant professor at the Aalto University, where I also obtained my doctoral degree. Before coming back to Aalto I was a postdoctoral scholar at the Mathematical Institute at the University of Oxford. My research area is the relatively new field of "network science" or "complex networks". This means that I'm interested in complex systems with a large number of elements that are interacting with each other in some non-trivial way and possibly leading to some emergent phenomena. Social systems are a good example: they consist of multiple elements (people) that are interacting with each other (social relationships) and lead to some very complex emergent behaviour (social groups, societies, conflicts, etc.). Other such complex systems include transportation systems, gene-regulatory systems in cells, ecological systems and many more. I see all of these systems as networks that can be studied with the similar sets of tools and theories.
-
Network science has been very successful in investigations of a wide variety of applications from biology and the social sciences to physics, technology, and more. In many situations, it is already insightful to use a simple (and typically naive) representation as a simple, binary graph in which nodes are entities and unweighted edges encapsulate the interactions between those entities. This allows one to use the powerful methods and concepts for example from graph theory, and numerous advances have been made in this way. However, as network science has matured and (especially) as ever more complicated data has become available, it has become increasingly important to develop tools to analyse more complicated structures. For example, many systems that were typically initially studied as simple graphs are now often represented as time-dependent networks, networks with multiple types of connections, or interdependent networks. This has allowed deeper and more realistic analyses of complex networked systems, but it has simultaneously introduced mathematical constructions, jargon, and methodology that are specific to research in each type of system. The concept of « multilayer networks » was developed in order to unify the aforementioned disparate language (and disparate notation) and to bring together the different generalised network concepts that included layered graphical structures. In this tutorial talk, I will introduce multilayer networks and discuss how to study their structure. Generalisations of the clustering coefficient for multiplex networks and graph isomorphism for general multilayer networks are used as illustrative examples.